Translate this page into:
Risk stratification in multiple myeloma – A review and update
*Corresponding author: Dr. Ritu Gupta, Department of Laboratory Oncology, All India Institute of Medical Sciences, New Delhi, India. Email: drritugupta@gmail.com
-
Received: ,
Accepted: ,
How to cite this article: Das N, Gupta R. Risk Stratification in multiple myeloma – A review and update. Ann Natl Acad Med Sci (India). 2024;60:120–30. doi: 10.25259/ANAMS-2023-1-7-(820)
Abstract
Multiple myeloma (MM) is a hematological malignancy of plasma cell origin with a prevalence rate of 1% and 10% of all cancers and hematopoietic malignancies, respectively. Though the median survival time has improved dramatically in the patients diagnosed with MM with the administration of novel therapeutic agents, the disease, by and large, remains incurable with frequent progression and relapses. In the recent past, an increased understanding of MM pathogenesis has opened facets for improved diagnosis, prognosis, and response assessment in patients diagnosed with MM. This review focuses on the various laboratory and clinical features used to stratify the MM patients into high vs. low-risk groups. Furthermore, it also highlights the role of artificial intelligence-based innovative research tools for risk stratification and prognostication in MM patients.
Keywords
Ethnicity-specific staging
Multiple myeloma
Staging
Survival
INTRODUCTION
Multiple myeloma (MM) lies at the malignant end of the spectrum of plasma cell proliferative disorder with variable clinical outcomes. The long-term survival of the patients with MM is variable and improvements have been observed over time with the use of novel therapeutic agents and stem cell transplantation.1,2 The marked heterogeneity in survival outcomes is attributed to clinical and laboratory parameters, which are used to categorize MM patients into different risk categories. Thus, the evaluation of various prognostic factors is essential to define as well as refine the therapeutic strategies to improve treatment outcomes. In the era of risk-adapted therapy, it is critical to identify high-risk patients to render effective treatment to achieve optimal response and good clinical outcomes. The current review deals with an appraisal of various aspects of risk stratification, including the historical staging systems, i.e. the Durie and Salmon Staging (DSS) and International Staging System (ISS), the current standard of care, i.e. the Revised International Staging System (R-ISS) and the latest artificial intelligence based staging systems like Modified Risk Staging System (MRSS), and Consensus-based Risk Stratification System (CRSS).3–7 This review also focuses on the new prognostic markers that are gaining relevance in patients treated with novel agents. Besides, the concept of dynamic monitoring and its significance in MM has also been addressed.
INCIDENCE
Multiple myeloma constitutes 1% of all cancers, 10%–15% of all hematological malignancies, and accounts for about 20% of all blood cancer-related deaths.8–10 Prevalence of MM is less in Asia and is about 1.1 per 1,00,000 individuals in contrast to the West where it accounts for 4.1 per 1,00,000 individuals.11 Similarly, the prevalence of MM in India is low as compared to Western countries, although it has been documented to be slowly increasing in urban India.11,12 The reported incidence of multiple myeloma in India is 1 per 1,00,000 individuals.13 The distribution of various plasma cell dyscrasias reported at our center from 2011-2018 is shown in Table 1. The age-standardized disability-adjusted life years (DALY) rate for multiple myeloma has markedly increased from 5.6 to 63.1 (28.2%) in India from 1990 to 2016.11
Disease | Number of patients | % |
---|---|---|
Multiple myeloma | 1371 | 82.7 |
Amyloidosis | 89 | 5.4 |
Plasmacytoma | 74 | 4.5 |
POEMS | 56 | 3.4 |
MGUS | 30 | 1.8 |
Smoldering myeloma | 13 | 0.8 |
PPCL | 11 | 0.7 |
POEMS, polyneuropathy, organomegaly, endocrinopathy, myeloma protein, and skin changes; MGUS, monoclonal gammopathy of undetermined significance; PPCL, primary plasma cell leukemia.
Multiple myeloma is slightly more prevalent in males as compared to females and the data from 27 population-based cancer registries in India during 2012–2014 showed that MM constitutes 1.19% of all cancers (95% CI: 1.14%–1.24%) with crude rates of 1.27 (95% CI: 1.20–1.35)/1,00,000 in men and 0.95 (95% CI: 0.89–1.02)/1,00,000 in women).14 The projected incidence of patients with MM in India for the year 2020 among males was 10,725 (cumulative risk = 1 in 465), among females was 7,756 (cumulative risk = 1 in 646), and for both sexes combined was 18,481 (cumulative risk = 1 in 541).15
PROGNOSTIC FACTORS FOR RISK STRATIFICATION IN MM
The identification of high-risk groups is relevant from a therapeutic and disease monitoring point of view. The risk stratification in MM has evolved over time to incorporate both clinical and laboratory parameters but the heterogeneity in presence and interpretation of the biomarkers is responsible for variable definitions of high-risk disease. The risk factors in MM can be broadly categorized into two , i.e. (1) patient-related factors and (2) disease-related factors.
-
Patient-related factors: Factors that are considered significant as individual patient-related factors are age, performance status, Frailty score, comorbidities, extramedullary disease, and renal failure.
Age: MM is rare in young individuals, with a reported frequency of 0.02%–0.3% in patients below 30 years of age.16,17 The median age of patients at diagnosis is approximately 65 years, with a third of patients over 75 years of age. However, in India, myeloma tends to occur in younger age groups with a median age of 56 years, signifying that MM occurs almost a decade earlier in India compared to the West.11 The recent AI-based modeling on Indian datasets has shown that MM patients aged 67 or higher have inferior survival outcomes.6,7
Performance status: Performance status is used to assess the daily living abilities of the patient to determine appropriate treatment, disease progression, and overall disease outcome. The various performance status tools considered in determining therapeutic options and clinical outcomes in myeloma include the Eastern Cooperative Oncology Group (ECOG) performance status and the Karnofsky Performance Status (KPS).18
Frailty score: Around a third of MM patients are above 75 years of age but account for 3/4th of myeloma mortality. Elderly patients benefit less from therapy due to drug-related toxicities and poor body physiology. A comprehensive additive scoring system (range 0–5), called Frailty score, combines age, comorbidities, cognitive and physical conditions and, identifies three groups, namely, fit (score = 0, 39%), intermediate fitness (score = 1, 31%), and frail (score ≥2, 30%). The other frailty scoring system included the Katz Activity of Daily Living (ADL) and the Lawton Instrumental Activity of Daily Living (IADL), estimating the physical and cognitive conditions, respectively.19 The International Myeloma Working Group (IMWG) frailty scale can predict survival and risk of toxicity from treatment in elderly and frail patients with MM.
Comorbidities: Various comorbid conditions influence the overall outcome of MM patients, and renal disease is one of the most important comorbid conditions.20 As MM is a disease of the elderly who tend to have higher comorbid conditions, several scoring systems were designed to assess the impact of comorbidities in this disease. The Charlson Comorbidity Index (CCI) and revised Myeloma Comorbidity Index (R-MCI) are the two most widely used scoring systems in MM.21 The CCI assesses a combination of 19 comorbid conditions and R-MCI considers only five parameters such as renal disease, lung disease, age, frailty score, and KPS. The other myeloma-specific comorbidity indices include ADL, the Mini-Mental State Examination (MMSE), and the quality-of-life 12-Item Short Form Health Survey Physical Composite Scale (SF-12 PCS).21 The various comorbidity parameters are a more objective and accurate way to assess the functional health status of myeloma patients and thus individualize treatment decisions. Engelhardt et al. in their study demonstrated that R-MCI is a reliable tool for risk prediction of overall survival (OS) and progression-free survival differences in a large patient cohort (n = 801).20 It has been shown that various co-morbid states such as renal, lung and KPS impairment, frailty, and age are significant risks for OS with median OS rates of 10.1, 4.4, and 1.2 years, respectively in fit, intermediate fit, and frail patients as per R-MCI index.20 In terms of risk prediction, the R-MCI and IMWG frailty scores generated comparable results. The 3-year OS rates were 90%, 74%, and 43% via the R-MCI for fit, intermediate-fit, and frail patients, respectively (P = 0.0006).20 Among other groups, the 3-year OS rates for fit and frail patients were 80% and 66% via the ADL (P = 0.0159), 78% and 48% via the MMSE (P = 0.0001), and 86% and 66% via the SF-12 PCS (P = 0.0091), respectively.21 Furthermore, it was found that R-MCI was proven to be the best predictor of survival in comparison to other comorbidity indices.20 Thus, the functional assessment of MM-specific comorbidity indices offers a precise evaluation of the prognosis and risk status in MM patients.
Extramedullary disease: Growth of malignant plasma cells beyond the bone marrow compartment is termed extramedullary (EM) myeloma. EM involvement in MM is an aggressive form of disease associated with poor survival outcomes.22,23 The reported incidence of EM disease was remarkably low before the positron emission tomography (PET) with computed tomography (CT) era, but with the advancement of imaging modalities, it is currently reported in up to 10% of newly diagnosed MM (NDMM) and 20% of patients with relapsed and refractory disease. 23-25 Available data on EM involvement also highlights the site dependency on outcome, with Central Nervous System (CNS) involvement having the worst outcome, followed by soft-tissue plasmacytomas and para-skeletal involvement.26
-
Disease-related factors: These factors can be broadly divided into (1) factors related to the disease load and (2) factors related to disease biology.
Factors related to disease load -– These include
Bone marrow plasma cells – Quantification of residual malignant plasma cells at the follow-up time point, commonly known as measurable residual disease (MRD) is a well-established post-treatment risk stratification tool in MM and recently incorporated in IMWG response criteria.27 MRD negative status is associated with survival benefits in all subsets of myeloma patients irrespective of demographic factors or treatment taken, as shown in a meta-analysis.28 In transplant recipients too, gradual reduction in malignant plasma cells at day 100% post-Autologous stem cell transplant (ASCT) is associated with improved PFS and OS.29 From a diagnostic time point perspective, it has been demonstrated that elevated malignant plasma cell % at diagnosis is associated with adverse clinical-pathological features, including anemia, elevated LDH levels, ISS3, RISS3, double hit and triple hit myeloma.30
Serum beta 2 microglobulin – Beta 2 microglobulin is ubiquitously present on the surface of all nucleated cells and is elevated in pathological conditions with high cell turnover. Serum beta 2M is a crucial parameter of the international staging system of multiple myeloma and is the global determinant of clinical outcome, predicting not only the prognosis but also the risk of progression from asymptomatic disease.4,31
Lactate dehydrogenase – Lactate dehydrogenase (LDH) is a biomarker of anaerobic glycolysis and thus invariably increases in various neoplasms and serves as an index of malignant transformation. Elevated LDH is associated with aggressive disease and high tumor burden, resulting in inferior progression-free survival (PFS) and overall survival (OS).32 Due to its crucial role in disease prognostication, LDH has been incorporated into the Revised International Scoring System (R-ISS) for myeloma.5
Monoclonal-protein (M-protein) -– A monoclonal spike on serum protein electrophoresis (SPE) is pathognomonic of MM except for true non-secretory variants. It is also elevated in other plasma cell dyscrasias (monoclonal gammopathy of undetermined significance – MGUS, Smoldering multiple myeloma (SMM)) and higher levels are associated with the risk of transformation to myeloma in the case of MGUS/SMM.33 The deleterious effect of the M-component is due to the deposition of immunoglobulins in renal tubules as well as the infiltration of various organs. It may cause hyperviscosity syndrome leading to cerebrovascular accidents. Due to consistently elevated levels of M-protein in MM and gradual fall with appropriate therapeutic intervention, M-protein plays a critical role in response evaluation and treatment monitoring in MM.
Serum-free light chains – Serum-free light chain (SFLC) assay has emerged as an important parameter for the diagnosis, monitoring, and prognosis of MM. MM cases are invariably associated with altered SFLC ratio (SFLCLR) with either kappa or lambda light chain excess.34 Several studies have demonstrated that SFLC measurement has a greater correspondence to tumor load especially at follow-up time points with small amounts of residual paraprotein.35 Furthermore, altered SFLCLR is an important risk factor with a higher probability of transformation to MM in patients with MGUS and SMM.36,37 As per the latest IMWG guidelines, the SFLC assay is particularly useful in oligosecretory/non-secretory variants, provided the SFLCR is abnormal, and the involved FLC level is ≥100 mg/L.
-
Factors related to disease biology – The disease biology of MM is influenced by multiple genetic alterations regulating the intrinsic biology of the plasma cells.38 With sequential acquisitions of genetic aberrations and the process of clonal evolution, patients develop a progression of disease and resistance to therapy.39,40. The primary genetic events in MM include IgH translocations and hyperdiploidy, while the secondary genetic events include copy number abnormalities, DNA hypomethylation, and acquired several somatic mutations.41
Primary genetic events: (i) IgH translocations – IgH translocations are considered as myeloma initiating events and are present in nearly half of the patients. They involve five chromosomal loci, 11q13, 6p21, 4p16, 16q23, and 20q11 which contain the CCND1, CCND3, FGFR3/NSD2, MAF, and MAFB oncogenes, respectively. Translocations t(4::14), t(14::16), and t(14::20) are associated with poor prognosis and are indicative of high-risk (HR) disease. On the other hand, t(11::14), t(6::14), and trisomies are considered to have been associated with standard-risk (SR) disease. (ii) Ploidy status – Hypodiploidy encompassing pseudodiploid, hypodiploid, and/or near-tetraploid variants carries poor prognosis.42,43 Hyperdiploidy in MM is seen in 50–60% of patients with frequent trisomies of chromosomes 3, 5, 7, 9, 11, 15, 19, and 2 and is associated with better survival outcomes.44
Secondary genetic events: Secondary cytogenetic abnormalities in MM are acquired over time and include gain(1q), del(1p), del(17p), del (13), RAS mutations, and translocations involving MYC. The deletion of tumor suppressor gene TP53 at 17p13 is observed in approximately 8–10% of NDMM and is associated with poor prognosis and poor survival.45 Both deletion at 1p and gain at 1q are associated with poor prognosis in MM, irrespective of the type of therapy given.46
Gene expression profiling - Evaluation of myeloma transcriptome with analysis of messenger ribonucleic acid (mRNA) and small non-coding RNAs has been used to assess its impact on outcome in MM.47 Gene expression profiling (GEP) is a tool to assess molecular heterogeneity using a much larger series of genes.48 In this context, the first whole genome sequencing by Chapman et al. revealed the presence of non-recurrent somatic alterations in MM.49 Following this, several NGS studies of the MM genome and exome demonstrated the spectrum of gene mutations associated with tumor progression 50 and have been reported most in KRAS (23%–26%), NRAS (20%–24%), and BRAF (4%–6%) genes that play a key role in MAPK pathway, nuclear factor kappa-light-chain-enhancer of activated B cells (NF-KB) signaling and FGFR3, TRAF3, and TP53.51 Thus, cytogenetic profiling in MM, which reflects the disease biology predicting survival, is of critical importance in risk stratification and overall prognosis. Figure 1 shows the cytogenetic risk stratification of MM.
Over the last decade, novel tools have been used to assess molecular heterogeneity of the genes involved in MM, which include techniques like real-time reverse transcriptase polymerase chain reaction (RT-PCR), array comparative genomic hybridization, single nucleotide polymorphisms, and next-generation sequencing (NGS). Shaughnessy et al. investigated the gene expression profile (GEP) of myeloma cells in NDMM and found a 70-gene signature linked to shorter durations of complete remission, event-free survival, and overall survival.52 Furthermore, in multivariate discriminant analysis, a 17-gene subset was identified that performed as well as the 70-gene model. Another large study published by Decaux et al. from the IFM group revealed 15-gene subsets involved in cell cycle progression and its surveillance and were identified to calculate a risk score that correlated with survival based on high-risk and low-risk groups.53 Several large clinical trials have shown that a high-risk GEP signature is associated with inferior treatment outcomes.54 Recently, a high-risk, “Double-Hit” group of NDMM was identified by genomic analysis using either (a) bi-allelic TP53 inactivation or (b) amplification (≥4 copies) of CKS1B (1q21) on the background of International Staging System III.55 Double-Hit MM was associated with dismal outcome with median PFS = 15.4 months; OS = 20.7 months. Though GEP is acclaiming an era of personalized medicine including risk-adapted therapy in MM, a great amount of prospective work is needed in this field.
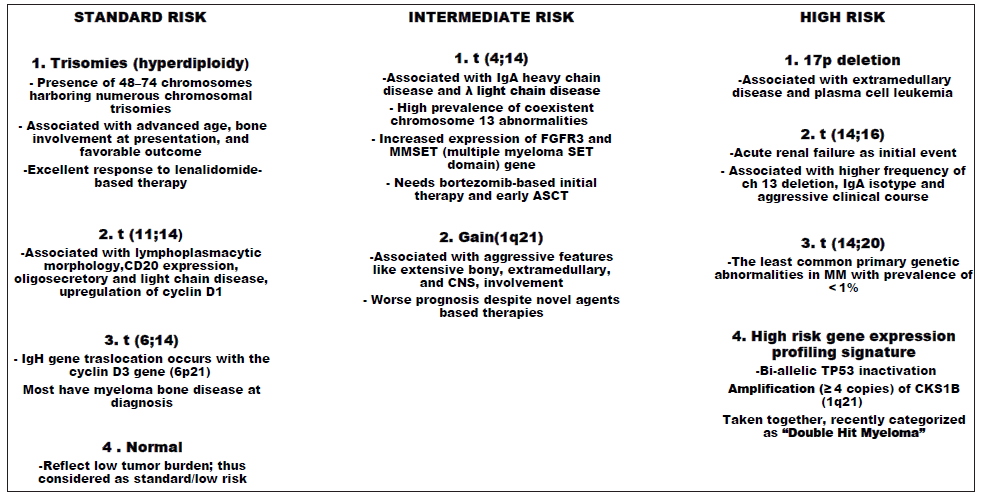
- Cytogenetics-based risk stratification in multiple myeloma. ASCT - Autologous stem cell transplant; CNS - Central nervous system; MM - Multiple myeloma
STAGING SYSTEMS
Based on various clinical, cytogenetics, and molecular markers defined above, several staging systems have been attempted since the mid-1970s to risk-stratify the patients diagnosed with MM.
-
The DS staging system: The first clinically recognized staging system for MM was introduced by Durie and Salmon in 1975, known as the Durie/Salmon (DS) system.3 The DS system was based on various clinical parameters that predicted myeloma cell tumor burden and included hemoglobin, serum calcium level, the type and quantity of monoclonal protein, and bone lesions. Creatinine level was further used to stratify the patients into good vs. poor risk groups [Table 2]. The major limitation of the DS staging system was the number of lytic lesions on routine radiographs, which were found to be observer-dependent and subject to variations. Another limitation of the DS system was that it was based on the utility of clinical parameters, which was considered a means to measure tumor mass rather than tumor biology-related factors like adverse cytogenetics. As a result, the DS staging system was found to be supplementing myeloma-associated diagnostic Calcium elevation; Renal insufficiency; Anemia; Bone abnormalities (CRAB) criteria viz hypercalcemia, renal dysfunction, anemia, and bone disease rather than truly predicting outcomes. Additionally, in the setting of novel therapeutic agents, the DS system was less predictive of treatment outcomes as novel agents more effectively reduced the myelomatous tumor burden.56 Thus, to ensure a precise and reproducible staging of newly diagnosed MM patients, several staging systems were proposed in the subsequent years;57,58 however, the DS staging system remained the most widely used staging system for NDMM for almost 25 years.
Table 2: Staging systems for multiple myeloma.Staging system DS-STAGING3 ISS4 RISS5 MRSS6 CRSS7 Parameters I II III I II III I II III 1 2 3 1 2 3 Age (in Years) <67 Fitting neither 1 nor 3 >67 <67 Fitting neither 1 nor 3 >67 Hb (g/dL) > 10 Fitting neither I nor III < 8 >12.3 ≤12.3 >12.3 ≤12.3 Calcium (mg/dL) <10.5 > 12 ≤11.3 >11.3 <11 ≥11 Albumin (g/dL) ≥3.5 < 3.5 - ≥3.5 Fitting Neither I nor III - >3.6 <3.6 >3.5 ≤3.5 Beta 2 microglobulin (mg/L) <3.5 3.5–5.5 >5.5 <3.5 ≥5.5 ≤4.85 >4.85 <4.78 ≥4.78 LDH Normal High Normal High M Protein (g/dL) • IgG < 5; IgA < 3 Fitting neither I nor III • IgG > 7; IgA > 5 24-h Urine Protein (g/24h) M-protein < 4 M-protein > 12 Imaging Normal bone structure on X-ray (scale 0), or solitary bone plasmactyoma Advanced lytic bone lesions (scale 3) Cytogenetic and molecular abnormalities *Standard risk Fitting neither1 nor 3 *High risk *Standard risk Fitting neither 1 nor 3 *High risk Serum Creatinine (g/dL) /eGFR A: serum creatinine < 2.0
B: serum creatinine ≥ 2.0
>48.1 Fitting neither 1 nor 3 ≤48.1 >48.2 Fitting neither 1 nor 3 ≤48.2 DS, Durie Salmon; ISS, International staging system; RISS, Revised International Staging System; MRSS, Modified Risk Staging System; CRSS, Consensus-based Risk Staging System ;LDH, Lactate dehydrogenase; eGFR, estimated glomerular filteration rate (*For standard and high-risk cytogenetic abnormalities, refer to Figure 1).
The ISS risk stratification: In 2005 Greipp et al. proposed an international staging system (ISS) based on two simple laboratory parameters of serum albumin and beta2-microglobulin.4 After the inception of the DS staging system, serum beta 2-microglobulin (Sβ2M) emerged as the single most reliable and powerful predictor of survival across various studies.59,60 In addition to β2M, several clinical biomarkers have been introduced as predictors of survival, Greipp et al.61-63 analyzed Sβ2M, serum albumin, platelet count, serum creatinine, and age by univariate and multivariate techniques, and found a combination of Sβ2M and serum albumin as the simplest and reproducible three-stage classification parameters [Table 2]. However, with the advent of cytogenetic abnormalities detected by iFISH as described above, the prognostication of MM patients was further refined and was later incorporated into the Revised International Staging System (RISS) as described below.
-
The RISS risk stratification: The fluorescent in-situ hybridization (FISH) cytogenetic revealed t (14; 16), t (14; 20), loss of p53 gene locus (del(17p), and monosomy 17 as a poor prognostic group across various studies64,65. Additionally, serum lactate dehydrogenase (LDH) level is found to be another key determinant of outcome in MM independent of conventional vs. novel therapeutic agents.66 LDH level above the normal reference range reflects a higher proliferation rate of the tumor mass associated with an aggressive course of the disease including extramedullary and extraosseous involvement.67 Thus, in 2015, Palumbo et al. combined ISS with cytogenetic features and serum LDH level and evaluated their prognostic relevance in NDMM, which they designated as the Revised International Staging System [Table 2].5 In this context, Gupta et al. also demonstrated nucleic acid-based tests (multiplex ligation-based probe amplification – MLPA and quantitative real-time polymerase chain reaction – qRT-PCR) as a resource as well as a cost-effective method to determine cytogenetic abnormalities as per R-ISS.68 Though the R-ISS staging system is a new risk stratification algorithm with an improved prognostic power compared with the ISS, the major limitation of RISS is the exclusion of host-related factors such as age, performance status, and comorbidities, which play an important role in defining patient prognosis. Another pitfall in RISS is the lack of interlaboratory standardization of FISH analysis and heterogeneous cutoff levels for LDH across the laboratories. Thus, in clinical practice, a better definition of MM risk groups is essential to provide more effective personalized therapies.
Modified risk staging system (MRSS): The novel therapeutic agents in survival data of RISS comprised predominantly of immunomodulatory drugs, however, with the advent of proteasome inhibitors, it is observed that few adverse cytogenetics like t (4;14) lost their poor prognostic relevance.69 Thus, from both clinical and research points of view, it is essential to characterize patients with poor clinical outcomes to develop effective therapies. In this context, Farswan et al. recently developed a machine learning-derived MRSS utilizing six clinical parameters, i.e., age, albumin, β2-microglobulin (β2M), calcium, estimated glomerular filtration rate (eGFR), and hemoglobin [Table 2].6 This artificial intelligence (AI)-based staging system performed better than ISS and RISS as a predictor of long-term survival on the MM Indian (MMIn) data set. Additionally, for the Multiple Myeloma Research Foundation (MMRF) repository, MRSS showed a superior survival outcome than RISS but a comparable survival rate with ISS.6 Thus, it is interesting to see the utility of this recently devised prognostication tool as a long-term predictor of survival across multiple prospective studies.
Consensus-based Risk Stratification System (CRSS): Another artificial intelligence (AI)-based staging system was also developed by the same group last year taking into consideration the ethnicity, designated as the Consensus-based Risk Stratification System (CRSS).7 Recently the role of ethnicity in the differential laboratory parameters was identified in the two independent cohorts of MMIn and MMRF patients with NDMM belonging to two separate ethnic groups. Furthermore, an AI-enabled risk stratification system was proposed incorporating the ethnicity-specific cut-offs of the laboratory parameters viz. albumin, Sβ2M, calcium, estimated glomerular filtration rate (eGFR), hemoglobin, and age along with high-risk cytogenetics. The rationale behind the development of such a staging system was to find an ideal risk staging system based on all the known adverse prognostic factors, including clinical, ethnic, and molecular aberrations. High concordance-index and hazard ratios revealed the superior performance of CRSS as compared to R-ISS. Thus, it was demonstrated that risk-stratification achieved by AI-assisted CRSS can better separate the patients into different risk groups as compared to R-ISS [Table 2].
DYNAMIC RISK STRATIFICATION
All staging systems described above are directed at risk stratification at the time of diagnosis, however, MM is a continuously evolving disease with changes in disease biology during the course of disease, responsible for possible relapse. There has been documentation of changes in risk factors at relapse.70 The evolving consensus is that, if a patient acquires high-risk features at relapse or progression, then that patient should be reclassified as having a high-risk disease. Therapy-related poor-risk features include progression while on therapy and short duration of response. Thus, in patients with relapsed disease, additional risk stratification criteria include the type of response and length of response to prior therapy. In this regard, Mayo Stratification for Myeloma and Risk-Adapted Therapy (mSMART) is a consensus opinion that considers genetically determined risk status and the various treatment strategies currently available.71 In 2007, Mayo Clinic introduced myeloma risk stratification or risk-adapted therapy as an evidence-based system that is constantly evolving and updated.
Response to therapy is one of the significant measures of dynamic risk assessment. Response to the first therapy has been shown to be associated with PFS and OS. We have already demonstrated that response after the first induction therapy was a predictor of PFS as well as OS.68 In post-transplant patients too, after ASCT, depth of response achieved is the most significant parameter of survival outcomes.29
The clinical parameter that is overlooked in most of the staging systems except DS staging is the utility of imaging as a prognostic tool. The number of bony lesions present in the DS system has already been considered prognostically insignificant due to low sensitivity as at least 30% destruction is required to visualize an intramedullary bone disease and its inability to detect extra-osseous lesions. There is a paucity of literature recommending the role of imaging studies in MM risk stratification. A study by Walker et al. showed that achieving magnetic resonance imaging (MRI) directed complete remission has a better prognosis than those with the presence of lesions.72 In another study, the prognostic value of Fluoro-deoxyglucose positron emission tomography (FDG-PET) and MRI was compared and FDG-PET at diagnosis was the only imaging modality significantly associated with inferior survival outcome.73 However, such studies depicting the role of imaging are limited, and thus a larger number of prospective studies are needed to demonstrate the utility of imaging as a prognostic parameter.
Measurable residual disease (MRD) assessment by flow cytometry or molecular techniques and its correlation with clinical outcomes in MM has been explored a lot in the last two decades.74 Another technique (complementary to molecular or flow cytometric assessment) to evaluate MRD is the use of imaging which can detect residual metabolically active focal lesions. In a recent study, MRD negativity is found to be associated with longer progression-free survival and overall survival and thus emerged as one of the strongest predictors of survival.30 The recent myeloma MRD data are supportive of MRD becoming a regulatory endpoint for drug approval in NDMM, based on which the IMWG recently revised the response criteria for myeloma and included MRD negativity as the highest degree of response to treatment.75 This emphasizes the fact that MRD is one of the single most important predictors of treatment outcome and thus should be incorporated in the MM risk stratification. Furthermore, MRD assessment also offers the advantage of dynamic risk stratification as it can be evaluated at any time during the disease, and based on MRD status, we can re-stratify the MM patients.
Risk stratification in resource constraint setting: Though a robust risk stratification system is mandatory for all myeloma patients for a timely institution of appropriate and adequate therapy, molecular studies and genomic profiling are available for a limited number of patients in a resource constraint setting. In this scenario, the use of various clinical and patient-specific factors becomes critical to annotate the patient as a good risk or vice versa. The MRSS system developed by Farswan et al. utilized six routinely evaluated parameters in MM, i.e., age, albumin, β2-microglobulin (β2M), calcium, estimated glomerular filtration rate (eGFR), and hemoglobin, to build a robust AI-based algorithm for survival predictions in MM.6 The MRSS which does not require genomic features for risk stratification was demonstrated to perform equivalent to R-ISS. Besides, determinants of MM disease burden viz LDH, high proliferation rates, extramedullary disease, hypercalcemia, high serum-free light chain ratio, plasmablastic disease, and plasma cell leukemia may also be used as predictors of outcome in a resource constraint setting. Thus, at the global level, it is imperative to strategize staging and risk prediction models for resource-constraint as well as resource-rich settings to ensure their implementation in real-life settings.
CONCLUSION
Though a number of individual risk factors have been identified so far, the general agreement is to use a system that incorporates multiple factors. However, none of the staging systems described so far is adequate to risk-stratify the MM patients in all scenarios. Thus, a comprehensive and robust risk stratification is needed in patients diagnosed with MM which takes into consideration not only laboratory-based parameters and cytogenetic abnormalities but also host-related factors like age, ethnicity, and co-morbidities. In this regard, AI-based staging systems like MRSS and CRSS are simple, reliable, and reproducible methods highlighting the role of machine learning models as an efficient prognostication tool that may be useful in planning better therapeutic strategies in MM patients. Furthermore, genomic features as well as measurable residual disease (MRD) though, emerged as the strong independent predictor of survival, have not been included in any of the risk stratification systems so far. Thus, the additional risk factors that are worthwhile to explore include the role of imaging, novel genomic signatures, including subclonal evolution during the course of therapy, and MRD evaluation for further refining of MM risk groups.
Ethical approval
Institutional Review Board approval is not required.
Declaration of patient consent
Patient’s consent not required as patients identity is not disclosed or compromised.
Financial support and sponsorship
Nil.
Conflicts of interest
There are no conflicts of interest.
Use of artificial intelligence (AI)-assisted technology for manuscript preparation
The authors confirm that there was no use of artificial intelligence (AI)-assisted technology for assisting in the writing or editing of the manuscript and no images were manipulated using AI.
REFERENCES
- Improved long-term survival in multiple myeloma up to the age of 80 years. Leukemia. 2014;28:1346-8.
- [CrossRef] [PubMed] [Google Scholar]
- multiple myeloma-effect of induction therapy on transplant outcomes. Clin Lymphoma Myeloma Leuk. 2021;21:80.e5-90.e5.
- [CrossRef] [PubMed] [Google Scholar]
- A clinical staging system for multiple myeloma. Cancer. 1975;36:842-54.
- [CrossRef] [PubMed] [Google Scholar]
- International staging system for multiple myeloma [published correction appears in J Clin Oncol 2005;23:6281. Harousseau, Jean-Luc [corrected to Avet-Loiseau, Herve]] J Clin Oncol. 2005;23:3412-20.
- [CrossRef] [PubMed] [Google Scholar]
- Revised international staging system for multiple myeloma: A report from International Myeloma Working Group. J Clin Oncol. 2015;33:2863-69.
- [CrossRef] [PubMed] [PubMed Central] [Google Scholar]
- AI-supported modified risk staging for multiple myeloma cancer useful in real-world scenario. Transl Oncol. 2021;14:101157.
- [CrossRef] [PubMed] [PubMed Central] [Google Scholar]
- Does ethnicity matter in multiple myeloma risk prediction in the era of genomics and novel agents? Evidence from real-world data. Front Oncol. 2021;11:720932.
- [CrossRef] [PubMed] [PubMed Central] [Google Scholar]
- Age and aging in blood disorders: Multiple myeloma. Haematologica. 2014;99:1133-7.
- [CrossRef] [PubMed] [PubMed Central] [Google Scholar]
- The burden of cancers and their variations across the states of India: the Global Burden of Disease Study 1990-2016. Lancet Oncol. 2018;19:1289-306.
- [CrossRef] [PubMed] [PubMed Central] [Google Scholar]
- Patterns of monoclonal gammopathy of undetermined significance and multiple myeloma in various ethnic/racial groups: Support for genetic factors in pathogenesis. Leukemia. 2009;23:1691-17.
- [CrossRef] [PubMed] [Google Scholar]
- Striking age differences of multiple myeloma (MM) diagnosis in patients of Indian and Pakistani descent in the United States compared to native countries. J Clin Oncol. 2017;35:e13070.
- [Google Scholar]
- Distribution of multiple myeloma in India: Heterogeneity in incidence across age, sex and geography. Cancer Epidemiol. 2019;59:215-20.
- [CrossRef] [PubMed] [Google Scholar]
- Cancer statistics, 2020: Report from National Cancer Registry Programme, India. JCO Glob Oncol. 2020;6:1063-75.
- [CrossRef] [PubMed] [PubMed Central] [Google Scholar]
- Multiple myeloma in patients younger than 30 years. Report of 10 cases and review of the literature. Arch Intern Med. 1996;156:1463-8.
- [CrossRef] [PubMed] [Google Scholar]
- SEER Cancer Statistics Review, 1975–2013. Bethesda, MD: National Cancer Institute; Apr, 2016. http://seer.cancer.gov/csr/1975_2013/, based on November 2015 SEER data submission, posted to the SEER website
- Impact of performance status on overall survival in patients with relapsed and/or refractory multiple myeloma: Real-life outcomes of daratumumab treatment. Eur J Haematol. 2020;105:196-202.
- [CrossRef] [PubMed] [Google Scholar]
- Geriatric assessment predicts survival and toxicities in elderly myeloma patients: An International Myeloma Working Group report. Blood. 2015;125:2068-74.
- [CrossRef] [PubMed] [PubMed Central] [Google Scholar]
- A concise revised myeloma comorbidity index as a valid prognostic instrument in a large cohort of 801 multiple myeloma patients. Haematologica. 2017;102:910-21.
- [CrossRef] [PubMed] [PubMed Central] [Google Scholar]
- Comparison of the prognostic significance of 5 comorbidity scores and 12 functional tests in a prospective multiple myeloma patient cohort. Cancer. 2021;127:3422-36.
- [CrossRef] [PubMed] [Google Scholar]
- Multiple myeloma with extramedullary disease: Impact of autologous stem cell transplantation on outcome. Bone Marrow Transplant. 2017;52:1473-5.
- [CrossRef] [PubMed] [Google Scholar]
- Expert review on soft tissue plasmacytomas in multiple myeloma: Definition, disease assessment and treatment considerations. Br J Haematol. 2021;194:496-507.
- [CrossRef] [PubMed] [Google Scholar]
- Soft-tissue extramedullary multiple myeloma prognosis is significantly worse in comparison to bone-related extramedullary relapse. Haematologica. 2014;99:360-4.
- [CrossRef] [PubMed] [PubMed Central] [Google Scholar]
- Standardization of 18F-FDG-PET/CT according to Deauville criteria for metabolic complete response definition in newly diagnosed multiple myeloma. J Clin Oncol. 2021;39:116-25.
- [CrossRef] [PubMed] [Google Scholar]
- Extramedullary disease in multiple myeloma: A systematic literature review. Blood Cancer J. 2022;12:45.
- [CrossRef] [PubMed] [PubMed Central] [Google Scholar]
- International Myeloma Working Group consensus criteria for response and minimal residual disease assessment in multiple myeloma. Lancet Oncol. 2016;17:e328-e346.
- [CrossRef] [PubMed] [Google Scholar]
- A Large meta-analysis establishes the role of MRD negativity in long-term survival outcomes in patients with multiple myeloma. Blood Adv. 2020;4:5988-99.
- [CrossRef] [PubMed] [PubMed Central] [Google Scholar]
- Graded depth of response and neoplastic plasma cell index as indicators of survival outcomes in patients with multiple myeloma following autologous stem cell transplant. Am J Clin Pathol 2022:aqac129.
- [CrossRef] [PubMed] [Google Scholar]
- Malignant plasmacytes in bone marrow detected by flow cytometry as a predictor for the risk stratification system of multiple myeloma. Cytometry B Clin Cytom. 2022;102:44-9.
- [CrossRef] [PubMed] [Google Scholar]
- Beta-2-microglobulin is an independent predictor of progression in asymptomatic multiple myeloma. Cancer. 2010;116:2188-200.
- [CrossRef] [PubMed] [Google Scholar]
- Prognostic value of serum lactic dehydrogenase (S-LDH) in multiple myeloma. Eur J Clin Invest. 1987;17:336-9.
- [CrossRef] [PubMed] [Google Scholar]
- Monoclonal gammopathy of undetermined significance and smoldering multiple myeloma: Emphasis on risk factors for progression. Br J Haematol. 2007;139:730-43.
- [CrossRef] [PubMed] [Google Scholar]
- International Myeloma Working Group guidelines for serum free light chain analysis in multiple myeloma and related disorders. Leukemia. 2009;23:215-24.
- [CrossRef] [PubMed] [Google Scholar]
- Diagnostic performance of quantitative kappa and lambda free light chain assays in clinical practice. Clin Chem. 2005;51:878-81.
- [CrossRef] [PubMed] [Google Scholar]
- Serum free light chain ratio is an independent risk factor for progression in monoclonal gammopathy of undetermined significance. Blood. 2005;106:812-7.
- [CrossRef] [PubMed] [Google Scholar]
- Immunoglobulin free light chain ratio is an independent risk factor for progression of smoldering (asymptomatic) multiple myeloma. Blood. 2008;111:785-9.
- [CrossRef] [PubMed] [PubMed Central] [Google Scholar]
- Characterizing The mutational landscape of MM and its precursor MGUS. Am J Cancer Res. 2022;12:1919-33.
- [PubMed] [PubMed Central] [Google Scholar]
- The genetic architecture of multiple myeloma. Nat Rev Cancer. 2012;12:335-48.
- [CrossRef] [PubMed] [Google Scholar]
- Branching clonal evolution patterns predominate the mutational landscape in multiple myeloma. Am J Cancer Res. 2021;11:5659-79.
- [PubMed] [PubMed Central] [Google Scholar]
- Genetic abnormalities in multiple myeloma: Prognostic and therapeutic implications. Cells. 2021;10:336.
- [CrossRef] [PubMed] [PubMed Central] [Google Scholar]
- Hypodiploidy is a major prognostic factor in multiple myeloma. Blood. 2001;98:2229-38.
- [CrossRef] [PubMed] [Google Scholar]
- Clinical impact of chromothriptic complex chromosomal rearrangements in newly diagnosed multiple myeloma. Leuk Res. 2019;76:58-64.
- [CrossRef] [PubMed] [Google Scholar]
- Chromosome abnormalities clustering and its implications for pathogenesis and prognosis in myeloma. Leukemia. 2003;17:427-36.
- [CrossRef] [PubMed] [Google Scholar]
- p53 Gene deletion detected by fluorescence in situ hybridization is an adverse prognostic factor for patients with multiple myeloma following autologous stem cell transplantation. Blood. 2005;105:358-60.
- [CrossRef] [PubMed] [Google Scholar]
- Genomic instability in multiple myeloma: Evidence for jumping segmental duplications of chromosome arm 1q. Genes Chromosomes Cancer. 2005;42:95-106.
- [CrossRef] [PubMed] [Google Scholar]
- Genome-wide identification of potential biomarkers in multiple myeloma using meta-analysis of mRNA and miRNA expression data. Sci Rep. 2021;11:10957.
- [CrossRef] [PubMed] [PubMed Central] [Google Scholar]
- Chromosome 1p21 deletion is a novel prognostic marker in patients with multiple myeloma. Br J Haematol. 2007;139:51-4.
- [CrossRef] [PubMed] [Google Scholar]
- Initial genome sequencing and analysis of multiple myeloma. Nature. 2011;471:467-72.
- [CrossRef] [PubMed] [PubMed Central] [Google Scholar]
- Genomic complexity of multiple myeloma and its clinical implications. Nat Rev Clin Oncol. 2017;14:100-13.
- [CrossRef] [PubMed] [Google Scholar]
- Molecular spectrum of BRAF, NRAS and KRAS gene mutations in plasma cell dyscrasias: Implication for MEK-ERK pathway activation. Oncotarget. 2015;6:24205-17.
- [CrossRef] [PubMed] [PubMed Central] [Google Scholar]
- A validated gene expression model of high-risk multiple myeloma is defined by deregulated expression of genes mapping to chromosome 1. Blood. 2007;109:2276-84.
- [CrossRef] [PubMed] [Google Scholar]
- Prediction of survival in multiple myeloma based on gene expression profiles reveals cell cycle and chromosomal instability signatures in high-risk patients and hyperdiploid signatures in low-risk patients: A study of The Intergroupe Francophone Du Myelome. J Clin Oncol. 2008;26:4798-805.
- [CrossRef] [PubMed] [Google Scholar]
- Gene expression profiling of plasma cells at myeloma relapse from tandem transplantation trial total therapy 2 predicts subsequent survival. Blood. 2009;113:6572-5.
- [CrossRef] [PubMed] [PubMed Central] [Google Scholar]
- A high-risk, Double-Hit, group of newly diagnosed myeloma identified by genomic analysis. Leukemia. 2009;33:159-70.
- [CrossRef] [PubMed] [PubMed Central] [Google Scholar]
- Prognostic factors and staging in multiple myeloma: A reappraisal. J Clin Oncol. 1986;4:80-7.
- [CrossRef] [PubMed] [Google Scholar]
- Prognostic features in the third MRC myelomatosis trial. Br J Cancer. 1980;42:831-40.
- [CrossRef] [PubMed] [Google Scholar]
- A new improved clinical staging system for multiple myeloma based on analysis of 123 treated patients. Blood. 1980;55:1011-9.
- [PubMed] [Google Scholar]
- Serum beta2 microglobulin and survival duration in multiple myeloma: A simple reliable marker for staging. Br J Haematol. 1983;55:439-47.
- [CrossRef] [PubMed] [Google Scholar]
- Prognostic value of pretreatment serum beta 2 microglobulin in myeloma: A Southwest Oncology Group Study. Blood. 1990;75:823-30.
- [PubMed] [Google Scholar]
- C-Reactive protein and beta-2 microglobulin produce a simple and powerful myeloma staging system. Blood. 1992;80:733-7.
- [PubMed] [Google Scholar]
- Plasma cell labeling index and beta 2-microglobulin predict survival independent of thymidine kinase and C-reactive protein in multiple myeloma. Blood. 1993;81:3382-7.
- [PubMed] [Google Scholar]
- A new staging system for multiple myeloma based on the number of s-phase plasma cells. Blood. 1995;85:448-55.
- [PubMed] [Google Scholar]
- A novel prognostic model in myeloma based on co-segregating adverse FISH lesions and the ISS: Analysis of patients treated in the MRC Myeloma IX trial. Leukemia. 2012;26:349-55.
- [CrossRef] [PubMed] [PubMed Central] [Google Scholar]
- Combining information regarding chromosomal aberrations t(4;14) and del(17p13) with the International Staging System Classification allows stratification of myeloma patients undergoing autologous stem cell transplantation. Haematologica. 2010;95:1150-7.
- [CrossRef] [PubMed] [Google Scholar]
- High serum lactate dehydrogenase adds prognostic value to the international myeloma staging system even in the era of novel agents. Eur J Haematol. 2010;85:114-9.
- [CrossRef] [PubMed] [Google Scholar]
- High serum levels of lactic dehydrogenase identify a high-grade lymphoma-like myeloma. Ann Intern Med. 1989;110:521-5.
- [CrossRef] [PubMed] [Google Scholar]
- Nucleic acid-based risk assessment and staging for clinical practice in multiple myeloma. Ann Hematol. 2018;97:2447-54.
- [CrossRef] [PubMed] [Google Scholar]
- Bortezomib plus dexamethasone induction improves outcome of patients with t(4;14) myeloma but not outcome of patients with del(17p) J Clin Oncol. 2010;28:4630-4.
- [CrossRef] [PubMed] [Google Scholar]
- Consensus recommendations for risk stratification in multiple myeloma: Report of The International Myeloma Workshop Consensus Panel 2. Blood. 2011;117:4696-700.
- [CrossRef] [PubMed] [PubMed Central] [Google Scholar]
- Management of newly diagnosed symptomatic multiple myeloma: Updated Mayo Stratification of Myeloma and Risk-Adapted Therapy (mSMART) Consensus Guidelines 2013. Mayo Clin Proc. 2013;88:360-76.
- [CrossRef] [PubMed] [Google Scholar]
- Magnetic resonance imaging in multiple myeloma: Diagnostic and clinical implications. J Clin Oncol. 2007;25:1121-8.
- [CrossRef] [PubMed] [Google Scholar]
- Comparative diagnostic performance of 1⁸F-FDG PET/CT versus whole-body mri for determination of remission status in multiple myeloma after stem cell transplantation. Eur Radiol. 2013;23:570-8.
- [CrossRef] [PubMed] [Google Scholar]
- Minimal residual disease evaluation in autologous stem cell transplantation recipients with multiple myeloma. Leuk Lymphoma. 2017;58:1234-7.
- [CrossRef] [PubMed] [Google Scholar]
- Role of MRD status in relation to clinical outcomes in newly diagnosed multiple myeloma patients: A meta-analysis. Bone Marrow Transplant. 2016;51:1565-8.
- [CrossRef] [PubMed] [PubMed Central] [Google Scholar]